Today, organizations across diverse sectors are accumulating vast quantities of data at an unparalleled scale. As aptly expressed, ‘data is the new oil’ and ‘data is the new currency,’ this complex myriad of information, if deftly utilized, can revolutionize business paradigms – driving innovation, steering informed decision-making, and fueling financial growth through monetized data products.
As digital data continues to grow at an unprecedented rate, organizations have remarkable opportunities to formulate strategies, implement decision-making, and drive innovation. Developing data products and finding strategic methods to monetize them enables organizations to harness their data powerfully. However, this process must be conducted ethically and within the boundaries of the law.
As organizations navigate the data-rich digital landscape, the key to monetizing this data lies in the strategic analysis, disciplined management, and creative formulation of data products – the conduits through which data transforms into monetary value.
So what are data products – “Data products are solutions (tools or insights) built to solve problems using technologies, tools, and methods that can learn from data. The creator/owner of this data can be the team tasked with developing data products, data generated by the organization’s operations, data bought externally, or procured through partnerships” Ref: https://openovation.co/thoughts-3/
Crafting Data-Driven Solutions: A Roadmap to Innovative Value Products
Creating data products means transforming your data into a practical and valuable form for others. This could include creating dashboards, generating reports, developing algorithms, establishing predictive models, or even turning your data into a SaaS product. These products generate value by providing insights, easing decision-making processes, and solving problems. The typical lifecycle of a data product is like any product lifecycle on an innovation curve. It’s an agile and non-linear process. However, breaking it down into the steps below should help understand it better as organizations identify opportunities and structure data product teams across their ecosystem. The data, the core ingredient for creating data products, innately provides fluidity to be creative in crafting value-delivery through design, lean and agile mindset.
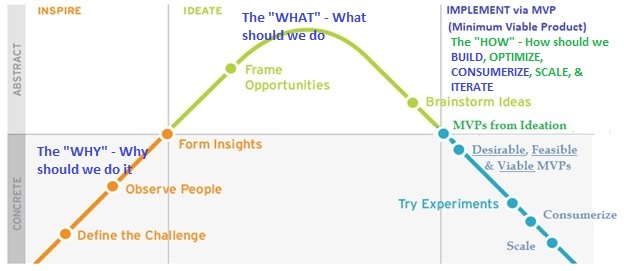
Ref: IDEO + ExperiencePoint: Design Thinking Innovation Process
Step 1: Understanding your data, business, and problems to be solved – the “WHY”
Organizations, regardless of the industry they operate in, are generating data in unprecedented volumes. When used appropriately, this data can serve as a valuable foundation for decision-making, strategy development, and innovation. Several factors contribute to the accumulation of data within organizations, including the growth of digital technologies, increased online interactions with customers, and an overall increase in digital processes within business operations.
Before organizations can capitalize on data products and monetization, it’s crucial that they first gain a deep understanding of the data they generate – “Understand your Data.” This encompasses inventorying all data assets, their source, and relevant information. These data assets could vary from customer demographics to transaction data, behavioral insights, and more – depending entirely on the business type.
The data product manager, one of the key roles in data product development, is intuitively aware of the customer’s problems through their interactions with the data evangelists & leaders, data scientists, customers, and business stakeholders and an understanding of the organization’s data assets. However, these problems may often need to be clarified, and product managers may have to deploy other techniques to understand the underlying problem and, therefore, the need and market for it. So, the first step in data product development is “understanding your data and problems.”
Step 2: Ideating on Potential Solutions (Ideas) – the “WHAT”
This step involves brainstorming and generating concepts for products or applications leveraging data to solve problems identified in Step 1. These solutions often involve data analytics, machine learning, AI, or other data-driven approaches. Here’s a breakdown of this ideation process:
Problem Understanding: Start by clearly understanding the problem or challenge you aim for, from Step 1, to address using data. Understanding the problem thoroughly will guide the ideation process. Example: The organization is facing massive customer churn for a subscription-based service.
Ideation of Data-Driven Solutions: Brainstorm various ways data can be leveraged to solve the defined problem. Consider the types of data needed, analytical approaches, and potential product features.
- Predictive Modeling: Create models to predict future outcomes based on historical data. Example: Build a machine learning model that predicts customer churning likelihood based on usage patterns, feedback, and demographics.
- Recommendation Systems: Suggest relevant items or actions to users based on their preferences and behavior. Example: Develop a recommendation engine that suggests personalized content or products to customers to improve engagement and satisfaction.
- Anomaly Detection: Identify unusual patterns or outliers in data, which may indicate potential issues or opportunities. Example: Design an anomaly detection system that alerts a manufacturing company of abnormal machine behavior, potentially indicating a quality issue.
- Optimization Algorithms: Develop algorithms to optimize processes or resource allocation based on data. Example: Create a customer support tool that optimizes the number of clicks from the customer, thereby improving user experience.
- Natural Language Processing (NLP): Analyze and derive insights from text data, facilitating sentiment analysis, topic modeling, and more. Example: Build a sentiment analysis tool to gauge customer sentiment from product reviews and social media, helping businesses understand public perception.
- Clustering and Segmentation: Group similar entities based on specific characteristics, enabling targeted strategies. Example: Cluster customers based on purchasing behavior to effectively tailor marketing campaigns and promotions.
Incorporating these ideation steps can help in generating a variety of potential data products, each aimed at addressing specific problems and creating value through data-driven approaches.
Step 3: Selecting and pursuing Desirable, Feasible, and Viable data product solutions from the backlog of ideas.
The selection step requires a structured approach to ensure alignment with business goals and market demands. The following considerations should lead to the most desirable, feasible, and viable data product portfolio for the problem at stake:
Market Demand and Desirability: Assess whether there is a significant demand for the data product. Example: Analyzing consumer surveys and online trends to identify a growing demand for an AI-powered language-translation tool.
Technical Feasibility: Evaluate whether the data product can be technically developed within existing technological capabilities and constraints. Example: Verifying if the required machine learning algorithms and data processing methods are achievable within the organization’s technical infrastructure.
Data Availability and Quality: Ensure that the necessary data for the product is available, relevant, and of sufficient quality. Example: Confirming the presence of reliable and diverse healthcare data for developing a predictive healthcare analytics tool.
Resource Availability: Assess the availability of required resources such as budget, skilled personnel, and time for development and maintenance. Example: Evaluating if the organization has the financial resources and experienced data scientists to create a real-time financial fraud detection system.
Business Model and Monetization Strategy: Determine a sustainable business model and strategy to monetize the data product effectively. Example: Developing a subscription-based model for an advanced data analytics platform targeted at businesses, ensuring consistent revenue streams.
Competitive Landscape: Analyze existing competitors and potential market entrants to understand the level of competition and differentiation opportunities. Example: Studying other ride-sharing apps before launching a new one to identify unique features that can give a competitive advantage.
Regulatory and Compliance Considerations: Assess the legal and regulatory requirements, ensuring compliance with data privacy and industry-specific regulations. Example: Confirm compliance with GDPR (General Data Protection Regulation) and HIPAA (Health Insurance Portability and Accountability Act) for a health-related data product.
Scalability and Adaptability: Verify that the data product can scale with increased usage and adapt to changing market needs and technological advancements. Example: Ensuring that an e-commerce recommendation engine can handle a surge in users during holiday seasons without declining performance.
By evaluating data product solutions based on these criteria and incorporating relevant examples, organizations can make informed decisions to pursue data products that are both feasible from a technical perspective and desirable and viable in the market, leading to successful outcomes and value creation.
Here’s an example of a criteria to prioritize data products for development
In this example, each data product is assessed and given a score of 10 for various criteria, representing their relative market demand, technical feasibility, data availability and quality, resource availability, business model, competitive landscape, regulatory compliance, and scalability/adaptability. The total score is calculated by summing the individual scores, providing a quantitative basis for prioritizing the development of data products.
Step 4: Blueprinting Success: Creating a Roadmap for Data Product Excellence – the “HOW”
Cross-pollinating from the “Design Thinking Innovation Process,” by this step, organizations should have a solid pipeline of desirable, viable, and feasible data product ideas to pursue. This step outlines the product roadmap and execution plan for each data product. This further enables the data product team to refine – the perceived value, cost/effort, and monetization potential.
Following are the core areas that are to be pursued with discipline and diligence at this step –
# Building the Dream Team: Key Roles in Data Product Organization Many roles are necessary for creating and managing data products. However, as organizations focus on data products, they would need to either hire or upskill in these data product roles –
- Data Engineers handle the collection, cleaning, and organization of data.
- Data Scientists analyze data and build models.
- Data Architects: Responsible for architecting and designing a solution that fits and scales within the required data and technological ecosystem. They are also responsible for data management, storage, and security. Data management includes the processes of data creation, storage, retrieval, and deletion, ensuring data’s high quality and availability. Using appropriate data storage solutions is critical to managing large databases. Several storage options are available, from traditional databases and data warehouses to cloud-based solutions. Each has different scalability and cost implications, so choosing the one that best suits the problem is crucial.
- Data Privacy and Security Analyst is another critical role. By abiding by privacy laws and guarding against data breaches, businesses retain the trust of their clients and avoid potential legal complications. Encryption, multi-factor authentication, and other security measures can protect your data from malicious attacks. Data Privacy and Security Analysts pay close attention to privacy and security measures to secure the data and insights.
- Data Product Managers oversee the product life cycle, gather user requirements, and ensure the products meet these needs). They ensure data products are user-friendly and usable. They also prioritize backlog features and product roadmap from user feedback and changes in the data, including new data. The data product manager is also responsible for identifying the best monetization strategy for the data product.
- Data Evangelists and Leaders are responsible for investing in infrastructure and tools that enable the smooth handling, analysis, and visualization of large volumes of data. They are also responsible for fostering a culture that promotes data-driven decision-making. Aside from the technical methodologies, creating a culture of data within the organization is paramount. This requires a shift in the mindset where data is accepted as an essential part of operations and strategic planning. Incorporating data-focused roles like data scientists, architects, and engineers into the organizational structure can also support this cultural shift.
# Data Collection and Integration Data Collection and Integration is like gathering puzzle pieces from different sources to complete a big picture. In data products, we need various information to make predictions or decisions, just like we need all the puzzle pieces to see the whole picture. Imagine planning a trip and needing weather forecasts, flight schedules, and hotel availability. Data Collection involves getting all this information from different places, like weather websites, airline databases, and hotel booking systems. Integration is like putting those puzzle pieces together so you can plan your trip effectively. In data products, it’s about combining data from various sources, like customer information, market trends, and product inventory, to make intelligent decisions, such as recommending the best vacation package for you. Example: “When you use a travel website to book a vacation, Data Collection and Integration help the website gather and combine data from airlines, hotels, and weather services to give you a complete picture of your trip, including the best flights and accommodations, ensuring you have a smooth and enjoyable journey.”
# Data Cleaning and Preprocessing Data Cleaning and Preprocessing is like preparing ingredients for a recipe—ensuring everything is fresh, clean, and ready to use. In data products, we start with raw data, which can be messy and have errors or missing pieces, just like fruits with peels or pits. Data Cleaning is like peeling, removing seeds, and washing the fruits to get them ready. We fix errors and fill in missing parts so that our ‘recipe’ (the model) can use the data effectively. Preprocessing is organizing these prepared ingredients, like chopping them into the right sizes for the recipe. For instance, imagine we’re predicting weather based on past data. Data Cleaning ensures our past weather data is accurate and complete while Preprocessing organizes it, so our weather prediction ‘recipe’ can work smoothly.” Example: “Think of data like a basket of fruits. Data Cleaning is sorting out the good, edible fruits and throwing away the rotten ones, while Preprocessing is cutting and arranging the good fruits so they are ready to use in a smoothie. Both steps ensure we have the best ‘ingredients’ for our predictions, just like having the best-prepared fruits for a delicious smoothie.
# Feature Engineering Feature engineering is like sculpting raw data into a more understandable form for our computer programs. Imagine you’re trying to predict house prices. Instead of just using the number of bedrooms, feature engineering involves creating new features like “price per square foot” or “neighborhood safety score.” These new features can provide more valuable information to our model, making it better at predicting prices accurately. In essence, feature engineering helps data products by transforming data into a shape that’s easier for the computer to work with, leading to more accurate predictions and valuable insights. This should further address
- is there value in data that can be offered “as is”
- do we need to create new features before value can be created for monetization or will there be a need to modify existing features for further analysis
# Model Development and Training Model development and training are like teaching a computer to learn from examples. Imagine you’re trying to predict whether it will be “rain-based” on past weather patterns. We collect data like temperature, humidity, and past rainfall to help the computer learn. The computer uses this data to create patterns and make predictions. It’s like learning to ride a bike—repeating and adjusting until you get it right. The computer does this automatically through various methods, creating what we call a ‘model.’ Think of it as an intelligent friend who learns from experiences and gives predictions based on what it learned. We then fine-tune this ‘smart friend’ to be as accurate as possible, ensuring it predicts rain (or anything else) as accurately as possible.
# Model Evaluation and Selection Model Evaluation and Selection is like choosing the best tool for a job. In data products, we have various ‘tools’ or models to predict things, like whether you’ll like a movie or if a product will sell well. We test these models like you’d test different hammers for a task to ensure our predictions are accurate. Imagine we have two hammers and want to see which drives nails better. We’d try them both, measure how well they work (accuracy), and pick the one that does the job best.
Similarly, we test different models in data products, like decision trees or neural networks, and choose the one that predicts outcomes most accurately. It’s like using the best hammer for each job, ensuring our predictions are as close to reality as possible. Example: “Imagine Netflix recommending movies to you. They use different ‘prediction tools’ (models) to guess what movies you might like. Model Evaluation and Selection helps them pick the best one, so you get the most accurate movie suggestions, making your movie night even better!”
# Model Deployment Model Deployment is like turning a recipe into a delicious meal everyone can enjoy. In data products, we need to make it work for real situations after we’ve created a ‘recipe’ for predicting things, like whether a customer will buy a product. It’s similar to taking a chef’s recipe and cooking it to serve it. For instance, if an online store wants to recommend products to shoppers, they’ve created a ‘recipe’ (a model) that suggests items. Model Deployment is putting that recipe to work on their website, so you see personalized recommendations in real time when you shop. It’s all about ensuring the model works smoothly and provides helpful suggestions to users. Example: “When you visit an e-commerce website like Amazon, Model Deployment shows you ‘Customers who bought this also bought’ recommendations. It takes the behind-the-scenes prediction ‘recipe’ and offers personalized product suggestions, making your shopping experience more enjoyable.
# Value Delivery, Monetization Strategy, and Pricing – Value Delivery, Monetization Strategy, and Pricing are critical aspects of introducing data products to the market. Value Delivery is about ensuring that the data product meets the specific needs and expectations of the users, providing meaningful insights or solutions. The monetization Strategy involves deciding how to generate revenue from the data product, whether through subscription models, one-time purchases, freemium offerings, or other methods. Pricing entails determining the optimal cost structure for the data product, considering factors like market demand, value proposition, competitive pricing, and affordability for the target audience. Example: “Consider a fitness app that tracks workouts and offers personalized training plans. Value Delivery is achieved by continuously improving the app to accurately track and provide tailored exercise regimens based on individual fitness goals. The Monetization Strategy might involve a combination of a free version with basic features and a premium subscription offering advanced workout analytics and personalized coaching for a monthly fee. Pricing decisions would be influenced by market research, competitor pricing, and ensuring that the subscription fee is attractive to fitness enthusiasts.
# Performance Monitoring “Performance Monitoring” for data products is crucial for maintaining optimal functionality and user satisfaction. Start by defining clear performance metrics, such as response time, error rates, and system availability. For instance, in an e-commerce recommendation engine, response time for generating product recommendations is a critical metric. Implement a monitoring system that continuously tracks these metrics and sets alert thresholds. For example, an alert is triggered if the response time exceeds a predefined threshold. Regularly analyze the data collected by the monitoring system to detect trends and patterns. For instance, a social media analytics tool might monitor user engagement rates and alert the team if engagement drops significantly. Use these insights to optimize the data product iteratively, ensuring it performs at its best and meets evolving user expectations.
# User Interface and Experience User Interface and Experience (UI/UX) in data products is like the design of a user-friendly app or a smooth, enjoyable ride in a well-designed car. When we create data products, it’s not just about the data and algorithms; it’s also about how people interact with them. Think of it as the dashboard in a car – it should be easy to understand, with clear signs and controls. In data products, UI/UX means making the information and features accessible and user-friendly. For example, a weather app shows the forecast with clear icons and an easy-to-read layout results from good UI/UX design. It helps you quickly get the information you need without any confusion. Example: “When you open a weather app and see a simple, intuitive display of the day’s weather, that’s an excellent User Interface and Experience at work. It makes checking the weather easy and enjoyable, just like a well-designed car dashboard makes driving a breeze.
# Scalability and Performance Scalability and Performance in data products is like ensuring a magic show can entertain a bigger audience without losing its wow factor. Imagine a magician wowing a small group; everyone gets amazed. Now, if that magic show can impress an entire stadium, that’s scalability. Data products are about ensuring our apps or websites can handle many people without slowing down. Like how a big stadium ensures everyone gets to see the magic, scalability ensures everyone can use an app smoothly. Performance is how fast and slick the magician performs. In data products, it’s how quickly a website loads or how fast it gives you recommendations. We want everything to be quick and impressive, no matter how big the ‘audience’ (users) is. Example: “Think of a social media app like Instagram. Scalability ensures that when a celebrity posts a photo and thousands of people rush to like it, the app doesn’t crash. Performance is how quickly you can scroll through your feed, view photos, and leave comments without delay. It’s like having a magic show that can dazzle a vast crowd while keeping the tricks sharp and exciting.
# Security and Compliance Security and Compliance are like your valuable possessions’ locks and safety measures. In data products, we deal with lots of information, just like you have personal belongings. We want to make sure this information is kept safe and used responsibly. Security is like a strong lock on your door, ensuring only the right people can access your house. Compliance is following the rules and guidelines, just as you follow traffic rules to ensure safety on the road. For example, if a health app collects your data to suggest exercise routines, they must keep that information safe (Security) and follow laws about data usage (Compliance). It’s about keeping things protected and following the right rules. Example: “Think of a health app that tracks your steps and heart rate. Security ensures your health data stays private and only accessible to you. Compliance ensures the app follows laws about health data privacy, keeping your information safe and handled correctly.
# Feedback and Iteration Feedback and Iteration is like improving a painting based on what others think about it. After we’ve created something like a recommendation system for data products, we want to know if it’s helpful. Feedback is like getting opinions on our painting. For instance, if an online streaming service suggests movies to you, they want to know if you liked the suggestions. Based on your feedback, they can improve the system by changing their recommendation process’s ‘colors’ or ‘brush strokes’ (Iteration). It’s all about fine-tuning and making improvements so that the data product becomes even more accurate and valuable for you. Example: “Imagine using Spotify, a music streaming app. When you like or dislike a song, it’s like giving feedback on the painting. Spotify takes this feedback and adjusts the type of music it recommends to you next time (Iteration). The more you ‘help’ improve the painting, the better it becomes at suggesting music you love!
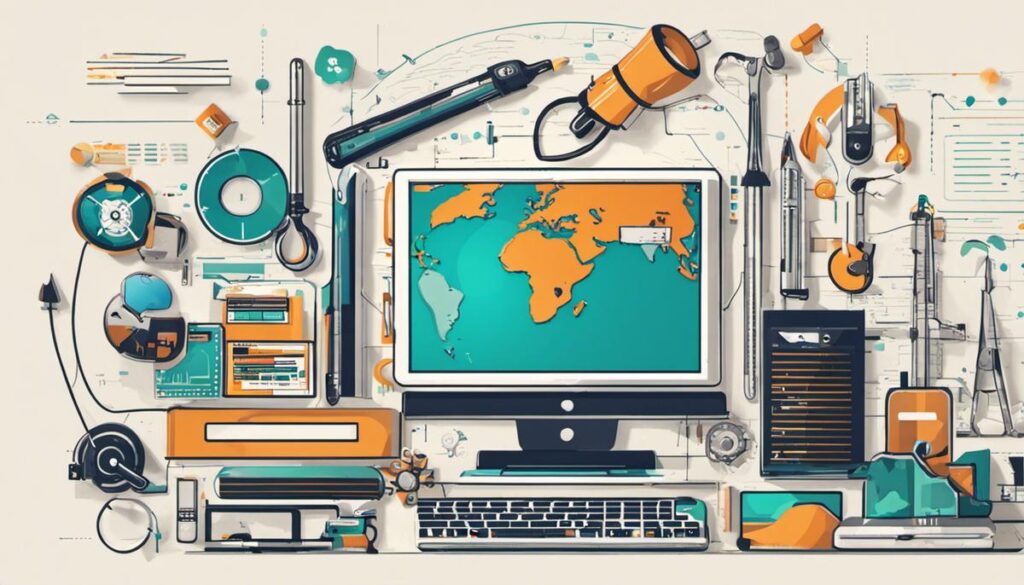
Unlocking Value: Data Monetization
Unless built to solve organization-specific problems, the data product is synergistically insufficient if it doesn’t contribute toward the bottom line. So comes the need for monetization strategies. Monetizing data necessitates a well-thought-out plan that leverages the right data products for profitable outcomes. This could involve selling aggregated datasets, charging for data services, or trading data for insights.
Businesses create immense data reservoirs through various activities such as standard operations, digital touchpoints, customer engagements, etc. This data pool can be processed and reconfigured into essential insights beneficial to internal decision-making processes and external revenue generation through direct or indirect monetization.
An organization can directly monetize its data by selling it to other companies that may need more resources or expertise to collect data themselves. This could be in the form of raw data or refined data that has been processed and analyzed to provide specific insights.
An example of direct data monetization includes selling customer behavior data to market research firms. These firms use this data to build accurate consumer profiles, which are used to guide businesses in their marketing strategies. Big data company Acxiom Corporation is a prime example of direct monetization. The company buys data from various sources, processes it, and then sells it to companies looking to target specific customer segments.
Direct data monetization could also involve giving access to your data for a subscription fee or combining your data with other providers to create premium products—however, consideration for the legal, ethical, and privacy implications while monetization is a must.
For example, a telecom company might choose to monetize its data by offering data bundles or selling insights about network usage to advertisers. Alternatively, a SaaS company might offer premium features that provide detailed analytics or enhanced functionality, transforming their data into a commodifiable product.
Indirect monetization involves using the data to provide customers with better services, products, or experiences, which results in enhanced revenue. This could be achieved through personalized marketing, improved service delivery, or launching new products based on insights from the data.
Netflix is an example of a company that monetizes its data indirectly. They use viewer data to understand what type of content users prefer. Then, they invest in creating similar content to keep the viewers hooked, maintain subscriptions, and attract new subscribers.
Tech giants like Google, Amazon, and Microsoft create data products like predictive analysis tools and artificial intelligence applications. For example, Google Photos identifies and labels images using machine learning, a form of data product.
The future of data monetization looks promising with advancements in AI and machine learning. Predictive analytics is one key trend to watch, where analytics will move from describing what happened to predict what will happen next. Moreover, there is a rise in creating data marketplaces where companies can sell and buy data in a secure and controlled environment. These platforms could redefine and streamline the process of direct data monetization.
Undeniably, data monetization carries enormous potential and presents gratifying opportunities for organizations. Yet, to truly capitalize on this wealth of data, establishments need to navigate a fine line between leveraging data, ethics, and safeguarding security and privacy.
Effective Data Management, Storage, and Security
Creating and monetizing data products also require effective data management, storage, and security. Data management includes the processes of data creation, storage, retrieval, and deletion, ensuring data’s high quality and availability.
Using appropriate data storage solutions is critical to managing large databases. Several storage options are available, from traditional databases and data warehouses to cloud-based solutions. Each has different scalability and cost implications, so choosing the one that best suits the problem is crucial.
Data security is another critical aspect. By abiding by privacy laws and guarding against data breaches, businesses retain the trust of their clients and avoid potential legal complications. Encryption, multi-factor authentication, and other security measures can protect your data from malicious attacks.
Ethical and Legal Considerations in Data Monetization
Alongside the potential revenue streams, organizations must also factor in ethical considerations and legal obligations when monetizing data. Data privacy laws, like the GDPR, demand that businesses respect users’ privacy and handle their data ethically. This can limit the data types sold and require consumer consent before their data is used. As a result, a strict data governance plan is crucial, outlining who has access to data and how it’s used, stored, and eventually deleted. The unprecedented ability to translate this data into lucrative data products opens new dimensions for monetization. Yet, this journey is full of ethical and legal challenges, requiring organizations to move forward while prioritizing transparency and trust carefully.
# Navigating Through Privacy Concerns – Privacy is one of the central concerns in data monetization. Building data products requires collecting, storing, and analyzing user data. However, a breach in data privacy can have severe legal implications and harm an organization’s reputation. Therefore, organizations need to obtain informed consent from individuals before collecting their data. They should also be transparent about data usage and allow individuals to opt out. Privacy policies should be clear and understandable and must meet regional and global standards for data protection, like GDPR in Europe.
# Addressing Data Ownership and Regulatory Restrictions – Data ownership is another complex issue in data monetization. Occasionally, organizations’ data is not theirs but sourced from third parties. Without explicit consent and user agreements, using such data can lead to legal issues. Therefore, it’s vital to verify data ownership and authenticity. Similarly, various industries have specific regulatory restrictions on data usage and monetization. For example, healthcare and finance follow stringent patient and customer data privacy rules. Hence, knowing and adhering to such regulations is crucial.
# Promoting Ethical Data Usage – Ethical data usage is fundamental to monetizing data. It should incorporate principles like fairness, accountability, and transparency. Using data ethically helps maintain public trust and avoid conflicts with consumers or regulators. For example, organizations should not use data to discriminate against specific groups or sell without the knowledge and consent of the individuals involved. Conducting regular data ethics training for employees to instill these values is recommended.
Despite the vast opportunities offered by data monetization, the journey is full of ethical and legal challenges that call for thoughtful navigation. A few best practices to pursue responsible data monetization should include –
- Regularly reviewing and updating privacy policies
- Maintaining transparency in the handling of data
- Taking ownership of data authenticity
- Adhering to the boundaries set by regulations.
- Advocating for Ethical Data Practices
By diligently adhering to these measures, organizations can not only stay clear of legal hindrances but also cultivate trust among their customers, thereby bolstering their brand image for the long haul.
Case Studies of Successful Data Monetization
# The Power of Data Monetization: Amazon – The monetization strategy of Amazon, the global e-commerce behemoth, illustrates successful data monetization. Amazon Web Services (AWS), a data product from the company’s stable, offers cloud computing services that makeup around 10% of Amazon’s total revenue. Leveraging the vast reserves of data amassed from its primary e-commerce operations, AWS delivers precious insights and services to organizations worldwide. Amazon’s use of data demonstrates how Amazon has expertly turned consumer and transactional data into a lucrative service provision.
# Data to Profit: Social Media Companies – Social media’s rise has fundamentally transformed how data is monetized. Platforms like Facebook and Instagram offer a free service to users but generate profits by selling targeted advertising opportunities to businesses. The role of user behavior data in this process is crucial. Every click, like, share, or comment is logged, analyzed, and packaged into data products businesses purchase to understand and reach their potential customers effectively.
# Healthcare Sector Utilizing Data – Data monetization has contributed enormously to care management and profit in the healthcare industry. Optum, a health services and innovation company, used analytics on patient records to predict patient hospitalization likelihood. Subsequently, preventive measures were implemented, reducing patient admissions and saving costs. This cutting-edge data product improved patient care and provided a substantial monetary benefit for the company.
# Technology Companies Leveraging Network Data – Telecom and technology companies leverage network data to create data products that improve business operations. For instance, Cisco deploys its network data into a Cisco Network Services Orchestrator product. This helps telecom companies streamline their network service lifecycles and bring services to market more quickly, generating significant revenue for Cisco.
# Data Monetization in the Automotive Industry – Telematics is becoming increasingly popular in the automotive industry. Companies utilize vehicular data to offer value-added services. Brands like BMW and General Motors have monetized their onboard vehicle data to provide vehicle health reports, roadside assistance, and concierge services, which are additional revenue streams.
The examples above show different strategies and methods to derive maximum utility from accumulated data while generating revenue. The crucial lesson is that data monetization is not limited to a specific industry.
Amalgamating the power of advanced technologies with the ubiquity of data, today’s organizations have the unprecedented opportunity to create diverse data products driven by user-centric needs while ensuring their ethical and legal usage. They can open new revenue streams and enhance their business growth by employing viable monetization strategies. As the case studies in this discourse exemplify, a strategic approach toward data has been the cornerstone of competitive advantage and sustainable success in the digital era. The call to action for enterprises is to tap into this potential, turning data from a dormant resource into an active strategic goldmine.