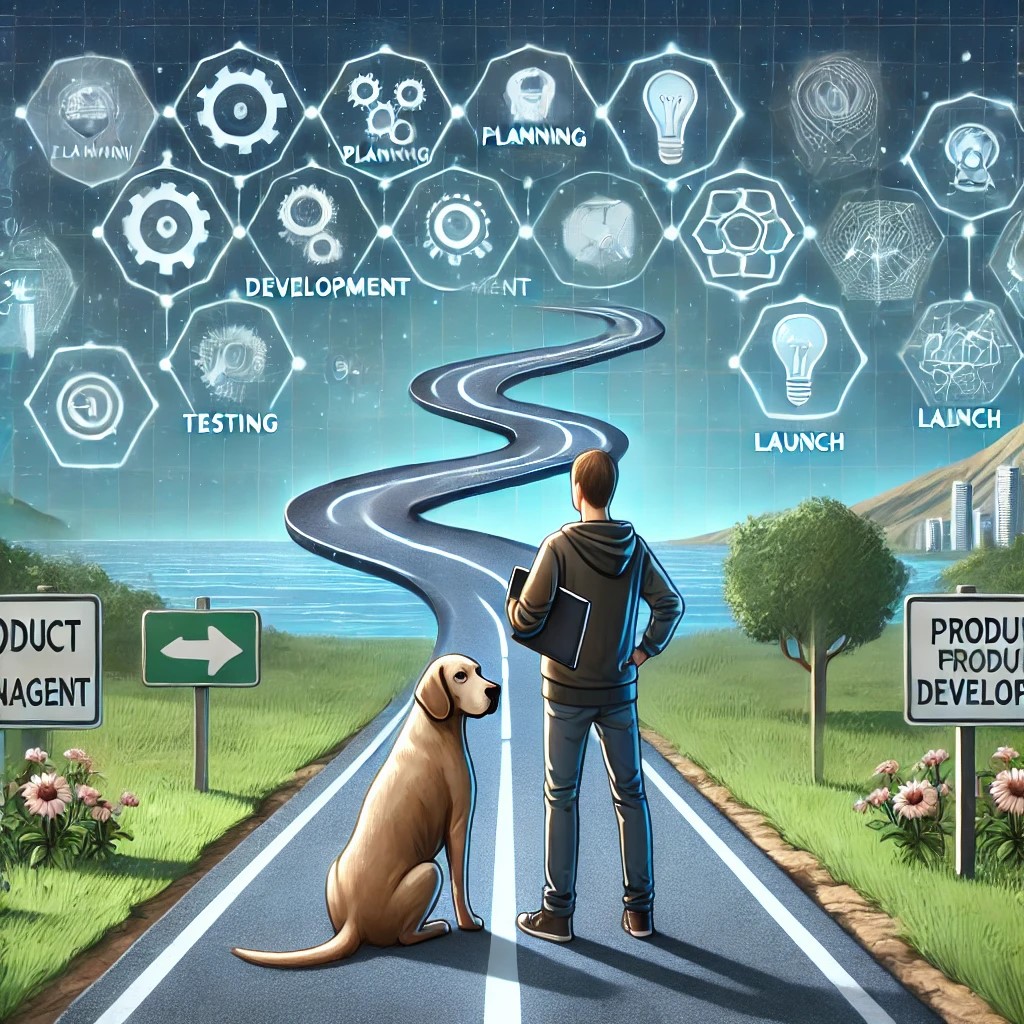
As someone driven by innovation, learning, brainstorming, observing, and building solutions, a deep dive into AI/ML/GenAI solutions continues to reveal the limitless potential of data that organizations have gathered over time. Data’s multidimensionality and the advanced techniques used to process and understand it today infuse more creativity than ever. Our only limitation is our imagination. Innovation possibilities through data are boundless, often emerging in situations that otherwise would have been dismissed just a few years ago.
For instance, combining unrelated datasets—such as stock market and healthcare data, dogs’ genetic data, and cuisine-related data—can lead to groundbreaking insights, conclusions, and AI products. Innovations are hidden in plain sight within the vast amounts of data available today, much of which is freely accessible. Platforms like Kaggle.com have made it easier for anyone to dive deeper and build solutions.
Personally and professionally, my inspiration continues growing as I study various datasets. The possibilities and hidden stories within these datasets are astonishing. It’s like connecting with a subconscious wisdom we always knew existed but didn’t know how or where to find it.
Aptly expressed, ‘data is the new oil’ and ‘data is the new currency. If properly utilized, this data can revolutionize business paradigms—driving innovation, steering informed decision-making, and fueling financial growth through monetized data products. The best part is with revolutionary tech and innovative practices around AI/ML, the cost of deriving information and, therefore, value is comparatively much lower and less complex.
As digital data continues to grow at an unprecedented rate, organizations have remarkable opportunities to formulate strategies, implement decision-making, and drive innovation. Developing AI-driven data products and finding strategic methods to monetize them enables organizations to harness their data powerfully. However, this process must be conducted ethically and within the boundaries of the law.
In this article, I have outlined how aspiring AI Product Managers may want to incept and build AI products via a framework outlined below.
Understanding AI-Driven Data Products
Data products are AI solutions (tools or insights) built to solve problems using technologies, tools, and methods that can learn from data. These solutions include creating dashboards, generating reports, developing algorithms, establishing predictive models, or even turning data into a SaaS product. These products generate value by providing insights, easing decision-making processes, and solving problems. The lifecycle of a data product is like any product lifecycle on an innovation curve – agile and non-linear. However, breaking it down into structured steps can help organizations identify opportunities and structure data product teams across their ecosystem.
Step 1: Understanding Your Data, Business, and Problems to Be Solved – the “WHY”
Organizations, regardless of their industry, generate unprecedented volumes of data. When used appropriately, this data can serve as a valuable foundation for decision-making, strategy development, and innovation. Several factors contribute to the accumulation of data, including the growth of digital technologies, increased online interactions with customers, and an overall increase in digital processes within business operations.
Before organizations capitalize on AI-driven data products and monetization, they must understand the data they generate— “Understand your Data.” This data exploration encompasses inventorying all data assets, their sources, and relevant information. Depending on the business type, data assets could vary from customer demographics to transaction data, behavioral insights, and more. With advancements in data management, including data mesh, visibility and exploration of varied datasets across the organization are now possible. This is an innovator’s paradise for AI products and solutions. By creatively mixing different colors (data), they could uncover a potential art (insights on problems to be solved) that no one would have thought of earlier.
Step 2: Ideating on Potential AI-Driven Solutions – the “WHAT”
This step involves brainstorming and generating concepts for AI-driven products or applications to solve identified problems. These solutions often involve data analytics, machine learning, AI, or other data-driven approaches. Here’s a breakdown of this ideation process:
- Problem Understanding: Start by clearly understanding the problem or challenge you aim to address using data.
- Ideation of Data-Driven Solutions: Brainstorm various ways data can be leveraged to solve the defined problem. Consider the types of data needed, analytical approaches, and potential product features.
- Predictive Modeling: Create models to predict future outcomes based on historical data.
- Recommendation Systems: Suggest relevant items or actions to users based on their preferences and behavior.
- Anomaly Detection: Identify unusual patterns or outliers in data, which may indicate potential issues or opportunities.
- Optimization Algorithms: Develop algorithms to optimize processes or resource allocation based on data.
- Natural Language Processing (NLP): Analyze and derive insights from text data, facilitating sentiment analysis, topic modeling, and more.
- Clustering and Segmentation: Group similar entities based on specific characteristics, enabling targeted strategies.
Step 3: Selecting and Pursuing Desirable, Feasible, and Viable AI Solutions
The selection step requires a structured approach to ensure alignment with business goals and market demands. The following considerations should lead to the most desirable, feasible, and viable AI product portfolio:
- Market Demand and Desirability: Assess whether there is a significant demand for the AI product.
- Technical Feasibility: Evaluate whether the AI product can be technically developed within existing technological capabilities and constraints.
- Data Availability and Quality: Ensure that the necessary data for the product is available, relevant, and of sufficient quality.
- Resource Availability: Assess the availability of required resources such as budget, skilled personnel, and time for development and maintenance.
- Business Model and Monetization Strategy: Determine a sustainable business model and strategy to monetize the AI product effectively.
- Competitive Landscape: Analyze existing competitors and potential market entrants to understand the level of competition and differentiation opportunities.
- Regulatory and Compliance Considerations: Assess the legal and regulatory requirements, ensuring compliance with data privacy and industry-specific regulations.
- Scalability and Adaptability: Verify that the AI product can scale with increased usage and adapt to changing market needs and technological advancements.
Step 4: Blueprinting Success – the “HOW”
Cross-pollinating from the “Design Thinking Innovation Process,” organizations should have a solid pipeline of desirable, viable, and feasible AI product ideas to pursue by this step. This step outlines the product roadmap and execution plan for each AI product, enabling the AI product team to refine the perceived value, cost/effort, and monetization potential.
Building the Dream Team: Key Roles in AI Product Development
Many roles are necessary for creating and managing AI products. Key roles include:
- AI Engineers: Handle the collection, cleaning, and organization of data.
- Data Scientists: Analyze data and build models.
- Data Architects: Responsible for architecting and designing solutions that fit and scale within the required data and technological ecosystem.
- Data Privacy and Security Analysts: Ensure the data is secure and compliant with privacy laws.
- AI Product Managers: Oversee the product life cycle, gather user requirements, and ensure the products meet these needs.
- Data Evangelists and Leaders: Foster a culture that promotes data-driven decision-making and invests in infrastructure and tools for handling, analyzing, and visualizing data.
Unlocking Value: AI-Driven Data Monetization
Monetizing AI-driven data products necessitates a well-thought-out plan that leverages the right data products for profitable outcomes. This could involve selling aggregated datasets, charging for data services, or trading data for insights.
Businesses create immense data reservoirs through various activities such as standard operations, digital touchpoints, customer engagements, etc. This data pool can be processed and reconfigured into essential insights beneficial to internal decision-making processes and external revenue generation through direct or indirect monetization.
- Direct Monetization: Selling data to other companies, offering data bundles, selling insights about network usage, or creating premium products with other providers.
- Indirect Monetization: Using data to provide better services, products, or experiences, resulting in enhanced revenue through personalized marketing, improved service delivery, or launching new products based on insights from the data.
Effective AI Data Management, Storage, and Security
Creating and monetizing AI-driven data products requires effective data management, storage, and security. Data management includes the processes of data creation, storage, retrieval, and deletion, ensuring data’s high quality and availability.
Ethical and Legal Considerations in AI Data Monetization
Alongside the potential revenue streams, organizations must factor in ethical considerations and legal obligations when monetizing AI data. Data privacy laws, like the GDPR, demand that businesses respect users’ privacy and handle their data ethically. This can limit the data types sold and require consumer consent before their data is used.
Case Studies of Successful AI Data Monetization
- Amazon: AWS leverages data to offer cloud computing services, making up around 10% of Amazon’s total revenue.
- Social Media Companies: Platforms like Facebook and Instagram generate profits by selling targeted advertising opportunities based on user behavior data.
- Healthcare Sector: Optum uses analytics on patient records to predict patient hospitalization likelihood, reduce patient admissions, and save costs.
- Technology Companies: Cisco uses network data to create products like Cisco Network Services Orchestrator, generating significant revenue.
- Automotive Industry: BMW and General Motors monetize vehicular data to offer value-added services.
By amalgamating the power of advanced AI technologies with the ubiquity of data, today’s organizations have the unprecedented opportunity to create diverse data products driven by user-centric needs while ensuring their ethical and legal usage. They can open new revenue streams and enhance their business growth by employing viable monetization strategies. As the case studies exemplify, a strategic approach toward data has been the cornerstone of competitive advantage and sustainable success in the digital era. The call to action for enterprises is to tap into this potential, turning data from a dormant resource into an active strategic goldmine.
As AI Product Managers lead their way in building AI solutions, here is what they could follow in the quantitative framework for AI Product Development
- Define Objectives and Key Results (OKRs):
- Clearly define what success looks like.
- Establish measurable vital results.
- Market Research and Needs Analysis:
- Identify target users and their pain points.
- Quantify the market size and potential demand.
- Data Collection and Preparation:
- Assess data availability and quality.
- Estimate the volume of data needed.
- Model Development and Validation:
- Select appropriate AI models.
- Quantitative metrics (accuracy, precision, recall, F1 score) were used to validate models.
- Integration and Deployment:
- Plan for scalability and integration with existing systems.
- Define performance metrics (latency, throughput).
- Monitoring and Optimization:
- Establish continuous monitoring for performance and accuracy.
- Use A/B testing to measure impact and optimize.
- ROI Calculation and Value Measurement:
- Calculate return on investment (ROI) using quantitative methods.
- Track key performance indicators (KPIs) post-deployment.
Real-Life Examples
- AI in E-commerce (Recommendation Systems):
- Objective: Increase sales through personalized recommendations.
- Key Results: Achieve a 15% increase in average order value within six months.
- Data Collection: User browsing and purchase history.
- Model Validation: Use metrics like click-through rate (CTR) and conversion rate.
- AI in Healthcare (Predictive Analytics):
- Objective: Reduce hospital readmissions.
- Key Results: Decrease readmission rates by 20% within a year.
- Data Collection: Patient health records and treatment outcomes.
- Model Validation: Evaluate using precision, recall, and ROC-AUC.
- AI in Finance (Fraud Detection):
- Objective: Minimize fraudulent transactions.
- Key Results: Detect 95% of fraudulent transactions with less than 1% false positives.
- Data Collection: Transaction data and user behavior.
- Model Validation: Monitor metrics like detection rate and false positive rate.